by Gino Terentim a Management 3.0 Facilitator
A few weeks ago I wondered how to start this article when I received a WhatsApp message saying that I had to talk about complexity. There was an episode in Honduras, where a bridge was built on the Choluteca River, and used to cross the river. Until then, everything was fine, except that a storm occurred and the river itself moved. The bridge is now on dry land, not being used for its intended purpose. The river is no longer there.
It was a perfect solution to the wrong problem.
This, for me, is one of the best examples of how an “obvious” solution is totally ineffective when it comes to complexity. The solution is perfect, as long as the problem does not change over time.
Reflecting on this, I asked myself:
How many problems are solved in our organizations in the same way, through a “copy and paste” format…and when the solution is finally ready, the problem has changed?
How many bridges, like Rio Choluteca’s are spread across our companies consuming resources and making organizations increasingly heavy because they have to carry the price of rework and waste?
The truth is that, as time goes on, the cycle of change becomes shorter and the pace at which new challenges are presented to organizations increases. I remember an article I read in Time magazine, called: The Year Man Becomes Immortal, about the accelerating pace of change and exponential growth in computing power that will lead to the Singularity.
While it took us 8000 years between the agricultural revolution and the industrial revolution, only nine years passed between the popularization of the internet and the sequencing of the human genome.
- How much time do our organizations have today to fully understand and understand a new problem before reacting?
- How much time do we have to gain enough knowledge about something so that we can establish a clear cause and effect relationship between a problem and a solution?
Decision-making in organizations is strongly based on the premise of repetition, predictability of the simplification of environments and reducing the whole to the sum of its parts.
Management 3.0, discusses that each element in a system is ignorant of the behavior of the system as a whole, and responds only to information that is available to it. This point is vitally important. If each element ‘knew’ what was happening to the system as a whole, all of the complexity would have to be present in that element.
In a school of fish, imagine if one was responsible for coordinating all of the movements and commanding the direction of the other fish and controlling whether everyone was executing as planned. He or she would most likely only notice the shark once several other fish had been eaten, because from his position he has only a partial view of the system and is unable to predict future behavior. The result can only be explained after it happens. Knowledge of past patterns is not enough (and may even be dangerous) to determine an answer in the future.
In a complex system, no one knows everything. Everyone only has one vision of the whole, but it is incomplete.
These two cases show the difficulty in using knowledge of the past to answer complex problems and ways of thinking. But, how do you know what is the best solution to a problem?
Here, we will look at two models for assessing complexity and at the end, a third (still incomplete and under construction), comparing them and highlighting their specific points.
The Cynefin Framework briefly explained
I’ll start with the Cynefin Framework, created by Dave Snowden, a former senior consultant at IBM, when he was director of the Institute for Knowledge Management around the early 2000s. During this period, he led the team that developed Cynefin, a framework for decision making.
The Cynefin framework classifies problems into three types of systems:
- Ordered
- Complex
- Chaotic
The three systems are subdivided into five domains: Clear, Complicated, Complex and Chaotic, those four require leaders to diagnose situations and then act with the appropriate response for the context.
The fifth domain, disorder, applies when there is no clarity as to which of the other domains is predominant. Usually, something in disorder is influenced by more than one domain. The complicated and clear domains are part of the same ordered system, emphasizing that the boundary between both is human and non-systemic, that is, the agent’s level of understanding and knowledge will determine whether something is complicated or clear.
#1: Clear Domain: Best Practices
It is characterized by the existence of a clear cause and effect relationship. The answer is known to all and unquestionable. In this domain, it is up to the leaders and managers to sense, categorize — according to a base or catalog of best practice — and respond. In this context, the effect will always be known and predictable.
Here the focus is on efficiency and management practices retain the fundamental characteristics of command and control, with top-down decisions, clear and very well-defined processes, with high predictability. The Clear domain has little ambiguity and therefore decisions can be easily delegated, and functions are automated. The network is less important than the hierarchy
#2: Complicated Domain: Good Practices
This is the domain of analysis and experts. Here, unlike what occurs in the clear domain, several solutions are possible for the same problem and although there is also a clear cause and effect relationship, it is not so obvious to the point that anyone can interpret it and react by simply categorizing it.
You need to analyze the data. Here, the presence of a specialist is necessary so that he/she can make an analysis in order to select which practice may be the best practice for a given situation. The leader or manager, in a complicated context, must sense, analyze and then respond. While the clear domain does not benefit from the use of networks, in the complicated domain, networks gain strategic importance, since the opinions of experts will be necessary to determine the best solution.
#3: Complex Domain: Emerging Practices
While a complicated context has at least one right answer, in a complex context this cause-effect relationship cannot be established. In this domain, the understanding needed in order to solve a problem does not come from the past, but from the future. While the previous domains establish rules and standards for making the environment fail-safe, here the role of the leader or manager will be to create a safe environment in which to fail. An environment for experimenting and the search for an emerging pattern. This is the domain of experimentation, the development of hypotheses, tests and the search for feedback to improve solutions. Emerging patterns can be perceived, but they cannot be predicted. In this context for decision making, a leader must establish a safe environment so that, through probing, he can recognize emerging patterns and get rid of those he does not want.
#4: Chaotic Domain: Novel Practices
The domain of quick responses: In a chaotic context, the search for patterns, good practices and the right answers is useless, since the relationship between cause and effect is impossible to establish. There are no patterns, and when we think that a new pattern has been identified, it will change. When a problem presents itself in the chaotic domain, the leader or manager should not waste time looking for patterns. You must act as soon as possible to try and stabilize the dramatic situation, then sense if the situation is in fact under control and only then respond by adding predictability (and reducing uncertainty) to the situation so that it can move to another domain.
In his book, Managing for Happiness, the foundation of Management 3.0, author Jurgen Appelo uses an approach that, at first glance, resembles the Cynefin Framework, however, when observed in more detail, it presents a different factor:
The observer’s perspective: The Y axis presents the level of difficulty to understand a given situation such as:
- Simple: Represents something extremely easy to understand
- Complicated: Something very difficult to understand
The X-axis shows the level of ability to predict the behavior of the situation:
- Orderly: it is totally predictable
- Complex: partially predictable & Chaotic: Totally unpredictable.
The Structure-Behavior Model of systems
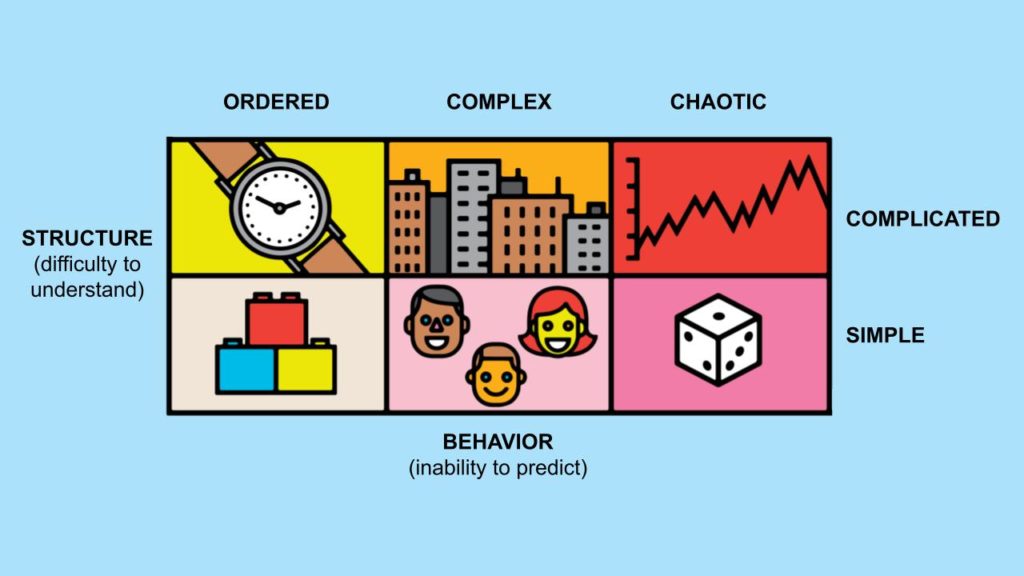
In this model, something can be considered simple or complicated, yet still be ordered, complex or chaotic. Everything will depend on two variables:
1: Level of simplification
2: Level of linearization
The level of simplification increases as something becomes easier to understand and the level of linearization increases as something becomes more predictable.
The Structure-Behavior Model (SBM) presents some similarities and differences in relation to the Cynefin Framework. The first difference is how to use them. SBM suggests an approach by categorization, in which the data is distributed in a pre-defined structure, that is, from an existing structure, the data is positioned in the quadrants. In Cynefin Framework, the domains have significant differences, as if they were on different planes. The border that separates the Clear from the Chaotic domain, for example, called a “zone of complacency”, reveals a region in which there is a “fall”. Cynefin is a sense-making structure, which emerges from existing data. It is the data that determine the structure and its limits.
The Cynefin Framework is used mainly to understand the dynamics of situations, in order to find the best decision-making process, helping to understand what is happening around us and, thus, enabling us to find the best answer to be adopted.
In addition to the visible similarities between the models, which bring with them the perspective of systems present in nature, another great similarity that makes them closer than distant is the “observer” view. Although the SBM makes use of categorization, when considering the level of difficulty in understanding about a given situation, the influence of the level of knowledge of the agents in the system is evident.
The example in the figure, the clock, in the complicated x ordered quadrant, is dependent and totally relative under this perspective. The operation of a watch, which is complicated for me, is absolutely obvious and simple for an experienced watchmaker. On the other hand, assembling a lego (simple-> ordered quadrant) can be an extremely complicated challenge for those who have never had contact with plastic blocks. In both cases, the level of knowledge present in the agent determines the quadrant in which the decision to be taken will be positioned.
Cynefin Framework vs Management 3.0 Structure-Behavior Model
An important point of difference between the two models is the concept applied to the types of systems
While SBM considers the level of predictability to be the determining factor for linearization, the Cynefin Framework, as detailed at the beginning of this article, separates systems using other criteria, such as attractors, boundaries and types of constraints. Chaos, for example, which contains an image of the stock exchange in the complicated-> chaotic quadrant of SBM, would conceptually be classified as Complex for the Cynefin Framework, as chaotic systems have no restrictions. The stock exchange, although unpredictable in the medium to long term, has some known patterns and clear restrictions, such as the hours of operation, currencies accepted, rules for investments, etc…
When the behavior of the stock exchange becomes totally unpredictable, someone “pulls the plug” and ends the operation, until the known restrictions (even small ones) are restored.
Something that caught my attention in relation to decision making and, especially, about the models and practices that I have been deepening in my studies over the last few years, is precisely the influence of the agent’s perception regarding his level of knowledge of the situation analyzed.
This reminds me a little of the Socratic paradox (I know that I know nothing) and the Dunning-Kruger effect — a phenomenon in which individuals who have little knowledge on a given subject believe they know more than others who have greater knowledge on the same topic. In other words, how can I be sure that something is really simple, if I am analyzing the phenomenon under the strong influence of my own knowledge (or lack thereof)?
I confess that this question has puzzled me for a long time, and it still puzzles me today.
About a year ago, researching the systems (ordered, complex and chaotic) and Johari’s Window, in order to see if anyone else suffered from the same dilemma, I came across an interesting article called: Cynefin framework and Johari window synergy with Risk Management. Although it is essentially an article on risk management, what caught my attention most was the comparison between Johari’s Window and the Cynefin Framework, which was exactly what I was looking for.
First a conceptual explanation: Johari’s window is a conceptual tool, created by Joseph Luft and Harrington Ingham in 1955, which aims to assist in understanding interpersonal communication and relationships with a group. Johari’s window application allows people to better understand their relationship with themselves and with other people.
Now, about the model. The window is divided into two axes: Me and the others. On each axis there is a quadrant related to the known and another to the unknown, so that a combination of 4 possible results is possible:
Johari’s Window Arena
This is an area where all the behaviors about which both I and others are aware are present. They are reciprocal and mutually known perceptions, that is, other people see the individual in the same way as the individual sees him/herself.
The Facade: Where are the behaviors I see about myself, but I do not allow others to see them. In this area, others see an image that does not reflect exactly who I am, and, for this reason, I must constantly take care to ensure that they do not perceive the “real me”.
Blind Spot: Where are the characteristics and behaviors that others perceive about me, but that I myself do not know and cannot perceive, that is, blind spots for me and visible to others.
Unknown: Where there are characteristics and behaviors that I do not know about my own personality and that others cannot perceive. Some of these unknown components may become aware of self-exposure (in a safe environment) and with a constant search for feedback. The change in one of the quadrants causes a change in the entire system.
Three Key Things to Highlight
#1: Asking for feedback: A movement established by accepting and encouraging the perception of others about ourselves, to identify how our behaviors are affecting them. This dynamic is as if we are seeing ourselves through the eyes of others. This makes the area that people know about me and I don’t know need shorter feedback loops, so that I can learn more about myself and, with that, migrate to another region.
#2: Disclosing and giving feedback: The movement through individual action, the “I”, offering feedback to others, identifying through their perceptions and feelings, how the behavior of others can affect me. Here I am the expert, and I need to give feedback so that people know more about me.
#3: Insights: The greater the self-exposure in a safe environment and the greater the amount of positive feedbacks, the stronger the vector towards Discovery, reducing the size of the unknown area. When we analyze the matrix formed by the Johari Window, there is a sudden desire to relate it to the domains of the Cynefin Framework, or to the quadrants of the Structure-Behavior Model. But I bravely resisted the temptation! I believe that each domain or quadrant can receive all the states of the matrix at a given moment and, precisely through blind spots, facades or unknown areas, both models retain in themselves an emerging property, a “perpetual innovation”, which allows us to constantly investigate and challenge them so that, little by little, we reduce the gap between a question and a good answer.
Excellent. Very nice insight especially the first half of the article. I was struggling to relate to the challenges which has triggered to create the framework and also scenarios where it can be deployed. While one can relate to the definition as given in 2nd part of the blog, it’s applicability or why this framework and where one can use it is nicely covered in first half and that is more crucial. Thanks for sharing.
Excellent exposure.
Clear and assertive. I was just thinking about translation the system ordered to Encomendado to Portuguese. I know as ordered system that it is obvious and complicated.
But this is nothing more than nomenclatures.
Cynefin is a wonderful framework that can help everyone in charge of decision making in our organizations. I think the use of Cynefin to determine what kind of problem (which domain) we are dealing with in association with the principles and tools of Management 3.0 are powerful resources to leaders and team prevail in times of content and fast changes.
Without a doubt, always look at the context of things, and treat problems, like pains each with their right medicine.
Great article my friend.
Regards